【AWS】bitflyer 自動注文(続き)
【きっかけ】
過去の記事参照
buffalokusojima.hatenablog.com
1. 使用するサービス
(AWS)
- Lambda
- IAM
- SSM
- SQS
(外部サービス)
2.概要
前回の続きになります。
buffalokusojima.hatenablog.com
今回は実際に自動注文を行う部分を説明します。最初に注文を手動で行い。注文IDをDynamoDBに格納し、約定したかを前回のLambdaで確認します。
約定確認のLambdaは前回作成した部分で、今回の説明範囲は最初の注文の部分です。主な機能は以前の記事に記載したbitflyerへの注文受付を参照してください。こちらをベースとしてます。
buffalokusojima.hatenablog.com
3. 実装
3-1. バックエンド Lambda側
const AWS = require('aws-sdk'); const ssm = new (require('aws-sdk/clients/ssm'))(); const request = require('request'); const sqs = new AWS.SQS(); const lambda = new AWS.Lambda(); const NORMAL_QUEUE_URL = '通常注文SQS'; const SPECIAL_QUEUE_URL = '特殊注文SQS'; AWS.config.update({region: 'ap-northeast-1'}); const ddb = new AWS.DynamoDB({apiVersion: '2012-08-10'}); exports.handler = (event, context, callback) => { if(!event.body){ console.log('No form data Found'); callback(null, { statusCode: 200, body: JSON.stringify({message: 'No form data Found'}), headers: { "Content-type": "application/json" } }); return; } var body = JSON.parse(event.body); console.log(body) const COIN_PAIR = body.coin_pair; const PRICE = body.price; const SIDE = body.side; const SIZE = body.size; const TYPE = body.type; const PARAMETERS = body.parameters; const ORDER_METHOD = body.order_method; // 追加項目 この値で自動注文を注文処理またはキャンセル処理する const MODE = body.mode; // 追加項目 キャンセル処理をするに当たっての該当の注文ID const PARENT_ID = body.parent_id; if(!TYPE && !PARAMETERS && !PRICE){ console.log("invalid order:",body); callback(null, { statusCode: 400, body: JSON.stringify({message: "invalid order"}), headers: {"Content-type": "application/json"} }); return; } if(COIN_PAIR != 'FX_BTC_JPY'){ console.log("invalid coin pair"); callback(null, { statusCode: 400, body: JSON.stringify({message: "invalid order"}), headers: {"Content-type": "application/json"} }); return; } if(MODE != 'insert' && MODE != 'delete'){ console.log("invalid mode:", MODE); callback(null, { statusCode: 400, body: JSON.stringify({message: "invalid order"}), headers: {"Content-type": "application/json"} }); return; } // 自動注文送信処理 DB操作までは以前と同様です。 if(MODE == 'insert'){ var queueBody = {autoOrder: true} var queueUrl; var groupId; if(!PARAMETERS){ if(!checkElement(body)){ console.log("Bad body:", body); callback(null, { statusCode: 400, body: JSON.stringify({message: "invalid order"}), headers: {"Content-type": "application/json"} }); return; } queueBody.product_code = COIN_PAIR; queueBody.child_order_type = TYPE; queueBody.side = SIDE; queueBody.price = PRICE; queueBody.size = SIZE; if(TYPE == 'STOP'){ queueBody.trigger_price = queueBody.price; queueBody.price = 0; } queueUrl = NORMAL_QUEUE_URL; groupId = "NORMAL_ORDER"; }else{ if(ORDER_METHOD != "SIMPLE" && ORDER_METHOD != "IFD"){ console.log("bad order method:"); callback(null, { statusCode: 400, body: JSON.stringify({message: "invalid order"}), headers: {"Content-type": "application/json"} }); return; } for(const parameter of PARAMETERS){ if(!checkElement(parameter)){ console.log("Bad body:", parameter); callback(null, { statusCode: 400, body: JSON.stringify({message: "invalid order"}), headers: {"Content-type": "application/json"} }); return; } parameter.product_code = COIN_PAIR parameter.condition_type = parameter.type; if(parameter.condition_type == 'STOP'){ parameter.trigger_price = parameter.price; parameter.price = 0; } } queueBody.order_method = ORDER_METHOD; queueBody.parameters = PARAMETERS; queueUrl = SPECIAL_QUEUE_URL; groupId = "SPECIAL_ORDER"; } // SQSを送る前にDBでデータ取得し、操作が入る getDatafromDynamoDB(callback) .then(function(data){ data = data.Items; // 既に注文済の場合は注文をしません。 if(data.find(d => d.coin_pair == COIN_PAIR || d.price == PRICE)){ console.log('Data Already Exists:', data); callback(null, { statusCode: 401, body: JSON.stringify({message: 'Data Already Exists:'+ data}), headers: { "Content-type": "application/json" } }); return; } // 通常注文の場合parameterが無いのでdb挿入用のパラメータ作成 var parameter; if(queueBody.parameters) parameter = queueBody.parameters[0]; else{ parameter = { product_code: COIN_PAIR, trigger_price: PRICE, side: SIDE, size: SIZE } } parameter.trigger_price = parameter.trigger_price.toString(); parameter.size = parameter.size.toString(); parameter.id ="newOrder"; parameter.history_data = [] putDataToDynamoDB(parameter, callback); sendSQS(queueBody, queueUrl, groupId, callback); }); // 自動注文キャンセル処理 }else if(MODE == 'delete'){ if(!PARENT_ID){ console.log("invalid parent id:", PARENT_ID); callback(null, { statusCode: 400, body: JSON.stringify({message: "invalid order"}), headers: {"Content-type": "application/json"} }); return; } var params = { product_code: COIN_PAIR, price: PRICE.toString() } // DBから該当のレコードを削除 deleteDataFromDynamoDB(params, callback); var payload = { coin_pair: COIN_PAIR, parent_id: PARENT_ID }; payload = JSON.stringify({body:JSON.stringify(payload)}); // 注文キャンセルLambdaを呼び出してキャンセル callLambda(payload, callback) .then(function(data){ if(data.StatusCode != 200){ console.log("Delete Error:", data); callback(null, { statusCode: 400, body: JSON.stringify({message: "Delete failed:", data}), headers: {"Content-type": "application/json"} }); return; } var body = JSON.parse(data.Payload).body; var message = body.message; if(message == ''){ message = "Delete Success" } callback(null, { statusCode: 200, body: JSON.stringify({message: message}), headers: {"Content-type": "application/json"} }); return; }) }else{ console.log('wrong requestbody'); callback(null, { statusCode: 401, body: JSON.stringify({message: 'wrong requestbody'}), headers: { "Content-type": "application/json" } }); return; } function getDatafromDynamoDB(callback){ return new Promise(function (resolve) { var params = { TableName: 'stop_check_bitflyer' }; // Call DynamoDB to add the item to the table ddb.scan(params, function(err, data) { if (err) { console.log("Error", err); callback(null, { statusCode: 401, body: JSON.stringify({message: err.toString()}), headers: {"Content-type": "application/json"} }); resolve(null); return; } resolve(data); }); }); } function putDataToDynamoDB(params, callback){ var params = { Item: { 'coin_pair' : {S: params.product_code}, 'price' : {N: params.trigger_price}, 'side': {S: params.side}, 'size': {N: params.size}, 'id': {S: params.id}, 'history': {L: params.history_data} }, TableName: 'stop_check_bitflyer' }; console.log(params) // Call DynamoDB to add the item to the table ddb.putItem(params, function(err, data) { if (err) { console.log("Error", err); callback(null, { statusCode: 401, body: JSON.stringify({message: err.toString()}), headers: {"Content-type": "application/json"} }); return; } console.log("Success"); params = params.Item; callback(null,{ statusCode: 200, body: JSON.stringify({message: 'PutItem Success: {coin_pair: ' + params.coin_pair.S + ' price: ' + params.price.N + ' side: ' + params.side.S +' size: ' + params.size.N + ' id: ' + params.id.S + ' history: ' + params.history.L }), headers: { "Content-type": "application/json" } }); return; }); } function deleteDataFromDynamoDB(params, callback){ var params = { TableName: 'stop_check_bitflyer', Key: { 'coin_pair' : {S: params.product_code}, 'price' : {N: params.price} } }; ddb.deleteItem(params, function(err, data){ if (err) { console.log("Error", err); callback(null, { statusCode: 400, body: JSON.stringify({message: err}), headers: { "Content-type": "application/json" } }); return; } console.log("Success"); params = params.Key; callback(null,{ statusCode: 200, body: JSON.stringify({message: 'DeleteItem Success: {coin_pair: ' + params.coin_pair.S + ' price: ' + params.price.N }), headers: { "Content-type": "application/json" } }); return; }); } function sendSQS(queueBody, queueUrl, groupId, callback){ var id=new Date().getTime().toString(); // SQS message parameters var params = { MessageBody: JSON.stringify(queueBody), MessageGroupId: groupId, MessageDeduplicationId: id, QueueUrl: queueUrl }; console.log(params) sqs.sendMessage(params, function(err, data) { var responseCode = 200; // response and status of HTTP endpoint var responseBody = { message: '' }; if (err) { console.log('error:', "failed to send message " + err); responseCode = 500; } else { console.log('data:', data.MessageId); responseBody.message = 'Sent to ' + queueUrl; responseBody.messageId = data.MessageId; } callback(null, { statusCode: responseCode, headers: { 'Content-Type': 'application/json' }, body: JSON.stringify(responseBody) }); }); } function callLambda(payload, callback){ return new Promise(function (resolve) { var params = { FunctionName: "注文キャンセルLambda名", InvocationType: "RequestResponse", Payload: payload } console.log(params); lambda.invoke(params, function(error, res){ if(error){ console.error(error); callback(null,{ statusCode: 500, body: JSON.stringify({message: error.toString()}), headers: {"Content-type": "application/json"} }); resolve(null); } resolve(res); }); }); } function checkElement(element){ if(element.type == 'MARKET' || element.type == 'LIMIT' || element.type == 'STOP'){ if(element.side != 'BUY' && element.side != 'SELL'){ console.log("invalid side"); return false; } element.price = Number(element.price); element.size = Number(element.size); if(isNaN(element.price) && element.type != 'MARKET'){ console.log("number invalid:", element.price); return false; } if(isNaN(element.size)){ console.log("number invalid:", element.size); return false; } return true; } return false; } };
3-2. フロントサイド
ほぼ以前紹介したものと同じです。
①見た目の部分
非常にシンプルにしています。
<body> <div class="displayOrder"> <div id="specialOrder"> <div id="stop" class="orderArea"> <div class="orderTitle">STOP</div> <br> <div class="detailArea"> <span> <label for="StopOrderNumber">Num: </label> <input type="text" id="StopOrderNumber"/> </span> <br> <span> <label for="StopOrderPrice">Price: </label> <input type="text" id="StopOrderPrice"/> </span> </div> <div class="decideOrder"> <input type="button" id="StopBuy" onClick="stopBuy()" value="BUY"/> <input type="button" id="StopSell" onClick="stopSell()" value="SELL"/> </div> </div> </div> </div>
②注文送信部分
STOP注文のみ実装してます。以前紹介したフロントサイドとほぼ同じです。
sendRequest関数に関しても以前を確認してください。
function stopBuy(){ var num = document.getElementById("StopOrderNumber").value; var price = document.getElementById("StopOrderPrice").value; if(!num || !price){ console.log("element empty"); return; } var parameters = [{ "type": "STOP", "size": num, "price": price, "side": "BUY" }]; var body ={ 'coin_pair': 'FX_BTC_JPY', 'parameters': parameters, 'order_method': 'SIMPLE', "mode": "insert", "price": price }; var data = { url: URL, method: 'POST', body: body } sendRequest(data); } function stopSell(){ var num = document.getElementById("StopOrderNumber").value; var price = document.getElementById("StopOrderPrice").value; if(!num || !price){ console.log("element empty"); return; } var parameters = [{ "type": "STOP", "size": num, "price": price, "side": "SELL" }]; var body ={ 'coin_pair': 'FX_BTC_JPY', 'parameters': parameters, 'order_method': 'SIMPLE', "mode": "insert", "price": price }; var data = { url: URL, method: 'POST', body: body } sendRequest(data); }
4. 終わりに
これで自動注文の大まかな実装が完了します。基本的に暴騰、暴落時の機会損失を軽減する為に存在します。
レンジ相場ではいたすらマージ消費を行ってしまいます。いつかここもレンジ相場の場合はなるべくスキャって
少しでも稼いで損失を減らすような仕組みを作ろうかと思います。
また、以前触れたDynamoDBの一貫性について、一貫性をある程度担保する方法もあるようですが、そもそもデータに不整合が起こるとしても
数秒くらいのトランザクション間になるそうです。
ですので、以前の記事の課題としてはDynamoDBが原因というよりかはLambdaまたは注文方法に原因がありそうです。
少しCloudWatch、LINE通知で見たところ、OCOの注文が原因がありそうです。
OCO注文は2つ同時に注文を出し、片方の注文が約定するともう片方の注文はキャンセルする注文です。
しかし、見たところ1秒程度で同時にどちらの注文の約定条件を満たしてしまうと、どちらも注文が約定してしまうようです。
例えば、以下のOCO注文があったとします。
STOP BUY 1200000
LIMIT BUY 1199000
現在の価格が1199500だとします。これが1秒など一瞬で1200000と1199000を行き来すると注文がどちらも約定します。
※改めて再現性があるような状態で注文して確認してみます。もしかしたらbitflyerのサーバの状態にもよるかもしれません。
恐らく、内部的にもAPI叩いて注文キャンセルを行うことでOCO注文を実現している可能性があります。
【AWS】bitflyer 自動注文
【きっかけ】
過去の記事参照
buffalokusojima.hatenablog.com
1. 使用するサービス
(AWS)
- Lambda
- DynamoDB
- IAM
- SSM
(外部サービス)
2.概要
実際に注文を行うLambdaの実装に関しては過去記事参照。また、ベースとなるLambdaである約定確認Lambdaに関しても過去記事参照。
途中で呼び出すLambdaとして注文一覧取得Lambdaも必要です。
buffalokusojima.hatenablog.com
buffalokusojima.hatenablog.com
buffalokusojima.hatenablog.com
仕組みとしては、約定通知を受けた際に、事前に用意したDynamoDB内で挿入した注文がなくなってあれば、約定されたと認識し、約定をした注文とは
逆のSTOP注文をします。それにより、価格がある程度、下がるまたは上がった際に損切りを自動かつ無限に行ってくれる仕組みになります。
つまりは、価格が暴騰、暴落した際に自身で決めた価格で確実に購入、売却はされる状態にあるということです。機会損失をなくすことに注力した実装になります。
3. 実装
3-1. DynamoDBの設定
stop_check_bitflyerという名でテーブルを作成しています。
主な設定としては以下になります。
・Primary partition key ・・・ coin_pair (String)
・Primary sort key ・・・ price (Number)
NOSQLなのでややこしいですが、主キーと考えていただいて結構です。基本的に同じ価格のItemは存在しない。
挿入しようとすると上書きになります。
また、現状あるデータを参考として、載せると以下のようになります。
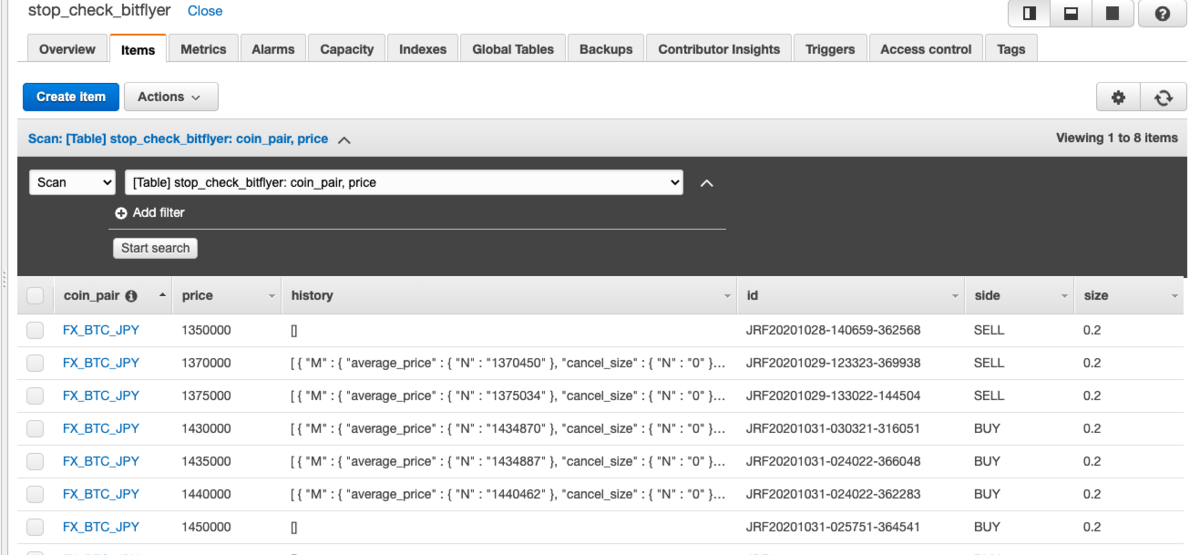
その他カラムに関してはプログラム内で追加しているので、そちらで説明します。事前いカラムを決めなくてもいいのがDynamoDBの特徴になります。
3-2. Lambda実装
ベースは過去記事で載せた約定通知Lambdaを改造したものになります。
以下、コード。
const AWS = require('aws-sdk'); const ssm = new (require('aws-sdk/clients/ssm'))(); const lambda = new AWS.Lambda(); const request = require('request'); const crypto = require('crypto'); const momentTimezone = require('moment-timezone'); const sqs = new AWS.SQS(); const ORDER_METHOD = 'OCO'; //const CONDITION_TYPE = 'STOP'; const STOP_PRICE_BIAS = 0.003; //STOP価格のバイアス const SPECIAL_QUEUE_URL = 'QUEUEのURL'; const queueUrl = SPECIAL_QUEUE_URL; const groupId = "SPECIAL_ORDER"; AWS.config.update({region: 'ap-northeast-1'}); const ddb = new AWS.DynamoDB({apiVersion: '2012-08-10'}); exports.handler = (event, context, callback) => { const id = process.env['id']; console.log('id: ' + id); getParameterFromSystemManager('bitflyer-keys',callback) .then(function(data){ const apikey = data.split(",")[0]; const sercretKey = data.split(",")[1]; var timestamp = Date.now().toString(); var method = 'GET'; var path = '/v1/me/getchildorders?product_code=FX_BTC_JPY&child_order_state=COMPLETED'; var afterID = '&after=' if(id){ path += afterID + id; } var text = timestamp + method + path; var sign = crypto.createHmac('sha256', sercretKey).update(text).digest('hex'); var option = { url: 'https://api.bitflyer.jp' + path, method: method, headers: { 'ACCESS-KEY': apikey, 'ACCESS-TIMESTAMP': timestamp, 'ACCESS-SIGN': sign, 'Content-Type': 'application/json' } } return sendRequest(option, callback); }).then(function(data){ if(data.response.statusCode != 200){ console.error("Error:",data.response); callback(null, { statusCode: data.response.statusCode, body: JSON.stringify({message: data.response}), headers: {"Content-type": "application/json"} }); return; } data = JSON.parse(data.body); if(data.length == 0){ console.log('No data Found'); callback(null,{ statusCode: 200, body: JSON.stringify({message: 'No data Found'}), headers: {"Content-type": "application/json"} }); return; } console.log(data) const dateTimeUtc = momentTimezone.tz(data[0].child_order_date.split(" ")[0], 'UTC'); const dateTimeJst = momentTimezone(dateTimeUtc).tz('Asia/Tokyo').format('YYYY/MM/DD HH:mm:ss'); const date = new Date(dateTimeJst); const toDay = new Date(momentTimezone(new Date()).tz('Asia/Tokyo').format('YYYY/MM/DD HH:mm:ss')); if(id == null && toDay.getTime() - date.getTime() > 180000){ process.env['id'] = data[0].id; console.log('Lambda Restarted'); callback(null,{ statusCode: 500, body: JSON.stringify({message: 'Lambda Restarted'}), headers: {"Content-type": "application/json"} }); return; } process.env['id'] = data[0].id; var message = '\n'; data.forEach(function(value){ const dateTimeUtc = momentTimezone.tz(value.child_order_date.split(" ")[0], 'UTC'); const dateTimeJst = momentTimezone(dateTimeUtc).tz('Asia/Tokyo').format('YYYY/MM/DD HH:mm:ss'); message += "[" + value.side + ": " + value.child_order_type + "]\n" + "Date " + dateTimeJst + "\n" + "price " + value.price + "\n" + "size: " + value.size + "\n" + "average price: " + value.average_price + "\n" + "executed size: " + value.executed_size + "\n" + "----------------------\n"; }); console.log(message); sendLine(message,callback); //ここから追加 //約定のデータをDynamoDB用に加工します。 var history = makeArrayforDynamoDB(data); //DynamoDBから自動注文のデータを取得して処理 getDatafromDynamoDB(callback).then(function(data){ if(data.Items.length == 0){ console.log("price check data not set"); callback(null, { statusCode: 200, body: JSON.stringify({message: "price check data not set"}), headers: {"Content-type": "application/json"} }); return; } const dbData = data.Items; //注文一覧Lambdaを呼び出す callLambda(callback).then(function(data){ var body = JSON.parse(data.Payload).body; data = JSON.parse(body).data //DynamoDBから取得したデータを元にfor文を回し、注文が約定しているか確認 for(var item of dbData){ //console.log(item.history.L) //注文一覧Lambdaから取得したデータ内にDynamoDB内にある注文IDと同じデータがあれば未約定としてスルー if(data.find(d => d.parent_order_acceptance_id == item.id.S)) continue; //STOP注文の作成 var side; var bias = Number(item.price.N) * STOP_PRICE_BIAS; if(item.side.S == 'BUY'){ side = 'SELL'; bias = -bias; }else if(item.side.S == 'SELL'){ side = 'BUY'; } //OCO注文として、最低限損失を少なくするようにする //何度も価格を行き来した際に、limit注文で損失を無くしていき、STOP注文で機会損失をカバーする var param1 = { product_code: item.coin_pair.S, condition_type: "STOP_LIMIT", side: side, size: item.size.N, price: item.price.N, trigger_price: item.price.N }; var param2 = { product_code: item.coin_pair.S, condition_type: "STOP", side: side, size: item.size.N, trigger_price: (Number(item.price.N) + bias).toString() } var parameters = [param1,param2]; console.log(parameters) var queueBody = { order_method: ORDER_METHOD, parameters: parameters, autoOrder: true } console.log(queueBody); //注文QUEUEを送信 sendSQS(queueBody, queueUrl, groupId, callback); var dbHistory = item.history.L dbHistory = dbHistory.concat(history) //console.log(dbHistory) param1.history_data = dbHistory; //DynamoDBに約定履歴や現状どのポジションで注文しているかをいれる putDataToDynamoDB(param1, callback); } }); }); }); function getParameterFromSystemManager(apikey_name, callback) { return new Promise(function (resolve) { var apikey = process.env[apikey_name]; if(!apikey || typeof apikey == undefined){ // Fetches a parameter called REPO_NAME from SSM parameter store. // Requires a policy for SSM:GetParameter on the parameter being read. var params = { Name: apikey_name, /* required */ WithDecryption:true }; ssm.getParameter(params, function(err, apikey) { if (err){ console.error(err.stack); callback(null,{ statusCode: 500, body: JSON.stringify({message: err.toString()}), headers: {"Content-type": "application/json"} }); resolve(null); return; } process.env[apikey_name] = apikey.Parameter.Value; resolve(apikey.Parameter.Value); }); }else resolve(apikey); }); } function sendLine(message, callback){ getParameterFromSystemManager('line-access-key', callback) .then(function(lineKey){ var option = { url: 'https://notify-api.line.me/api/notify', headers: { 'Content-Type': 'application/json', 'Authorization': 'Bearer ' + lineKey }, method: 'POST', form :{ message: message } }; return sendRequest(option,callback); }) .then(function(data){ if(data.response.statusCode != 200){ console.error(data) } callback(null, { statusCode: data.response.statusCode, body: data.body, headers: {"Content-type": "application/json"} }); }); } function makeArrayforDynamoDB(array){ if(!array || array.length == 0){ return; } var newArray = [] for(var item of array){ var newItem = {} Object.keys(item).forEach(function (key) { if(typeof(item[key])=="number") newItem[key] = {N: item[key].toString()} else if(typeof(item[key]=='string')) newItem[key] = {S: item[key]} }); newArray.push({M: newItem}); } return newArray; } function getDatafromDynamoDB(callback){ return new Promise(function (resolve) { var params = { TableName: 'stop_check_bitflyer' }; // Call DynamoDB to add the item to the table ddb.scan(params, function(err, data) { if (err) { console.log("Error", err); callback(null, { statusCode: 401, body: JSON.stringify({message: err.toString()}), headers: {"Content-type": "application/json"} }); resolve(null); return; } resolve(data); }); }); } function putDataToDynamoDB(params, callback){ console.log(params.id) if(!params.id){ params.id = ''; } var params = { Item: { 'coin_pair' : {S: params.product_code}, 'price' : {N: params.trigger_price}, 'side': {S: params.side}, 'size': {N: params.size}, 'id': {S: params.id}, 'history': {L: params.history_data} }, TableName: 'stop_check_bitflyer' }; console.log(params) // Call DynamoDB to add the item to the table ddb.putItem(params, function(err, data) { if (err) { console.log("Error", err); callback(null, { statusCode: 401, body: JSON.stringify({message: err.toString()}), headers: {"Content-type": "application/json"} }); return; } console.log("Success"); params = params.Item; callback(null,{ statusCode: 200, body: JSON.stringify({message: 'PutItem Success: {coin_pair: ' + params.coin_pair.S + ' price: ' + params.price.N + ' side: ' + params.side.S +' size: ' + params.size.N + ' id: ' + params.id.S + ' history: ' + params.history.L }), headers: { "Content-type": "application/json" } }); return; }); } function sendSQS(queueBody, queueUrl, groupId, callback){ var id=new Date().getTime().toString(); // SQS message parameters var params = { MessageBody: JSON.stringify(queueBody), MessageGroupId: groupId, MessageDeduplicationId: id, QueueUrl: queueUrl }; console.log(params) sqs.sendMessage(params, function(err, data) { var responseCode = 200; // response and status of HTTP endpoint var responseBody = { message: '' }; if (err) { console.log('error:', "failed to send message " + err); responseCode = 500; } else { console.log('data:', data.MessageId); responseBody.message = 'Sent to ' + queueUrl; responseBody.messageId = data.MessageId; } callback(null, { statusCode: responseCode, headers: { 'Content-Type': 'application/json' }, body: JSON.stringify(responseBody) }); }); } function callLambda(callback){ return new Promise(function (resolve) { var params = { FunctionName: "注文一覧Lambdaのファンクション名", InvocationType: "RequestResponse", //Payload: payload //リクエストパラメータはないのでいらない } lambda.invoke(params, function(error, res){ if(error){ console.error(error); callback(null,{ statusCode: 500, body: JSON.stringify({message: error.toString()}), headers: {"Content-type": "application/json"} }); resolve(null); } resolve(res); }); }); } function sendRequest(option, callback){ return new Promise(function (resolve) { request(option, function(error, response, body){ if(error){ console.error(error); callback(null,{ statusCode: 500, body: JSON.stringify({message: error.toString()}), headers: {"Content-type": "application/json"} }); resolve(null); } var data = {response, body} resolve(data); }); }); } };
4. 課題
DynamoDBの特徴として、RDBMSと違って、データの一貫性が保証されず、データの上書きと参照で相違が出る可能性があります。
というのも、価格が上下し、自動注文を繰り返していると、誤注文のような事象が稀に見られ、100%安全な注文とは言えません。
また、Lambdaの再起動時に環境変数が初期化することによる、約定データ大量取得にも原因があると思われます。
いずれ問題解決したり、LmabdaのリスタートやDynamoDBのデータ特徴に関して何かわかれば記事にしていこうかと思います。
【AWS】CloudformationでApiGatewayをデプロイ
1. 使用するサービス
(AWS)
- CloudFormation
2. 概要
前回の続きで、前回は初回のApiGatewayのデプロイを行いました。ですが、前回のままだと、ApiGatewayのリソースの更新までしか行いません。
ApiGatewayをデプロイまでを行う為にはCloudformationのApiGatewayの命名を変える必要があります。
#ApiGatewayのデプロイの設定 #ここをユニークな名前にする ApiGatewayDeployment1: Type: AWS::ApiGateway::Deployment Properties: RestApiId: Ref: RestApi Description: "apigateway deployment" #パラメータのステージ名を使用するよう設定 StageName: !Ref Stage
参考:
medium.com
【AWS】 CloudformationでAPI Gateway Lamda環境構築
1. 使用するサービス
(AWS)
- CloudFormation
2. 概要
前提として、S3に静的コンテンツがある前提です。今回の説明ではCloudformationのテンプレートでApiGatewayとLamdaのRestfulな環境をデプロイまでするとこまでを説明します。
以下、テンプレートのソースを載せます。
AWSTemplateFormatVersion: '2010-09-09' Transform: AWS::Serverless-2016-10-31 Description: Outputs the status #パラメータ設定 #Resources内で使用するパラメータを事前にこちらで定義することができます。 #Deploy前にCloudDeploy内で設定した値が入ります。 Parameters: Stage: Default: dev Type: String AllowedValues: [dev, test] #リソース設定 #実際に利用するサービスの設定を書きます。今回はApiGatewayにLambdaを直接書き込んで設定する方式をとります。 #別でMethodとして切り分けることも可能です。 Resources: #RestfulなApigatewayの設定 RestApi: Type: AWS::ApiGateway::RestApi Properties: Body: info: version: '1.0' title: !Ref 'AWS::StackName' #以下にパスの設定を記述していきます。例ではAPIのURL/helloを叩いた時の挙動を記述してます。 #基本的にコピペで大丈夫かと思います。CORSにも対応してます。 #パスを追加する際に変更する箇所にコメント入れておきます。 paths: /hello: #メソッドの種類になります。ここ以下は全てPOSTで大丈夫です。統合関係はPOSTだからだそうです。。。 get: responses: '200': description: "200 response" schema: $ref: "#/definitions/Empty" headers: Access-Control-Allow-Origin: type: "string" x-amazon-apigateway-integration: httpMethod: POST type: aws #実際にデプロイするURIのarnを記述します。変更する箇所としてはLambdaのarnになります。 uri: !Sub >- arn:aws:apigateway:${AWS::Region}:lambda:path/2015-03-31/functions/${HelloWorldFunction.Arn}/invocations responses: default: statusCode: "200" responseParameters: method.response.header.Access-Control-Allow-Origin: "'*'" passthroughBehavior: "when_no_match" httpMethod: "POST" type: "aws" options: x-amazon-apigateway-integration: type: mock schema: $ref: "#/definitions/Empty" requestTemplates: application/json: | { "statusCode" : 200 } responses: default: statusCode: '200' responseTemplates: application/json: | {} responseParameters: method.response.header.Access-Control-Allow-Origin: '''*''' method.response.header.Access-Control-Allow-Headers: '''Content-Type,X-Amz-Date,Authorization,X-Api-Key,X-Amz-Security-Token''' method.response.header.Access-Control-Allow-Methods: '''OPTIONS,POST''' consumes: - application/json summary: CORS support responses: '200': headers: Access-Control-Allow-Origin: type: string Access-Control-Allow-Methods: type: string Access-Control-Allow-Headers: type: string description: Default response for CORS method produces: - application/json definitions: Empty: type: object title: Empty Schema swagger: '2.0' #ApigatewayからLambdaを呼び出す設定 HelloWorldFunctionApiPermissiondev: Type: AWS::Lambda::Permission Properties: Action: lambda:InvokeFunction Principal: apigateway.amazonaws.com #下記にある呼び出したいLambdaを設定 FunctionName: !Ref HelloWorldFunction #Lamdaの設定 HelloWorldFunction: Type: AWS::Serverless::Function Properties: Handler: index.handler Runtime: python3.8 CodeUri: ./HelloWorld #使用したいRoleのArnを記述。今回は下で設定しているRoleを設定 Role: !GetAtt HelloworldRole.Arn #Roleの設定 HelloWorldRole: Type: 'AWS::IAM::Role' Properties: AssumeRolePolicyDocument: Version: "2012-10-17" Statement: - Effect: "Allow" Principal: Service: - "lambda.amazonaws.com" Action: - "sts:AssumeRole" #使用したいポリシーを記述 ManagedPolicyArns: - arn:aws:iam::aws:policy/service-role/AWSLambdaBasicExecutionRole MaxSessionDuration: 3600 Path: "/" #ApiGatewayのデプロイの設定 ApiGatewayDeployment: Type: AWS::ApiGateway::Deployment Properties: RestApiId: Ref: RestApi Description: "apigateway deployment" #パラメータのステージ名を使用するよう設定 StageName: !Ref Stage #デプロイのURiを設定 Outputs: RestApiUri: Export: Name: RestApiUri Value: !Sub "https://${RestApi}.execute-api.${AWS::Region}.amazonaws.com/${Stage}/"
以上で、CloudDeployでデプロイするとApiGatewayのRestfulな環境がデプロイされます。
Lambda内のコードを変更してデプロイ時でも更新されて指定された環境にデプロイされます。
【Deep Learning】Keras-yolo3のパッケージ化
Keras-yolo3の簡単なパッケージ化の例を載せておきます。
フォルダ構成は以下のようになっていて、各種フォルダからsrc内のKerasYolo3を呼び出すイメージになります。
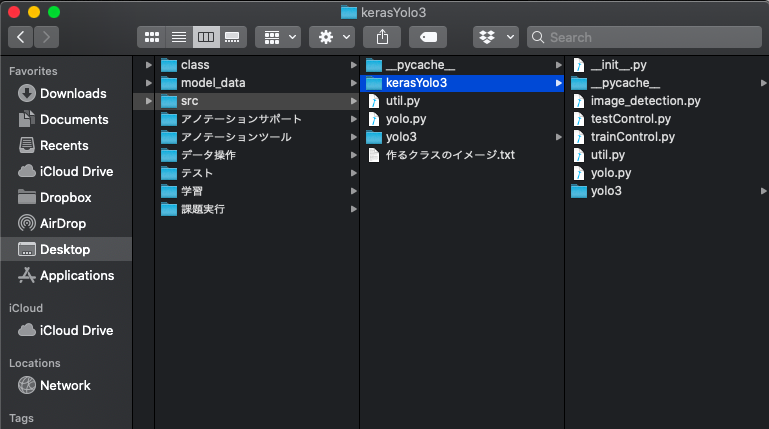
KerasYolo3フォルダないで__init__.pyファイルを作成し、以下のように記述します。
from src.kerasYolo3.image_detection import ImageDetector
これにより、KerasYolo3をパッケージとして認識され、import KerasYolo3をした際に、image_detectionモジュールもimportされるようになります。
以下、image_detectionモジュールです。簡単なYoLO呼び出しモジュールになります。
import colorsys import os,sys from timeit import default_timer as timer import numpy as np from keras import backend as K from keras.models import load_model from keras.layers import Input from PIL import Image, ImageFont, ImageDraw """ call yolo modules from yolo folder here """ from .yolo import YOLO class ImageDetector(): def __init__(self, model_path, classes_path): try: if not os.path.isfile(model_path): print('model path does not exist') return None if not os.path.isfile(classes_path): print('class path does not exist') return None self.yolo = YOLO(model_path=model_path, classes_path=classes_path) except Exception as e: print('Open Model Error:', e) return None # detect image function def detect_image(self, img_path): try: image = Image.open(img_path) except Exception as e: print('Open Image Error:', img_path, e) return None, None else: r_image, result = self.yolo.detect_image(image) return r_image, result def close_session(self): self.yolo.close_session()
srcと同じフォルダ内で実行ファイルを作成します。以下のようにモジュール呼び出しをしてみます。
import src.kerasYolo3 as yolo imageDetect = yolo.ImageDetector("model_data/種類モデル/type-model.h5", "class/種類クラス/class.txt")
実行すると、クラスから上手くインスタンスが作成されることがありあります。
【Deep Learning】アノテーションの効果
画像認識AI作成に当たって行うであろう、アノテーションについて、アノテーションの仕方によるAIの画像認識の仕方の違いについて記述する。
1. 概要
1枚の画像に対して、複数認識対象がある画像に対して、ある1対象のみをアノテーションする場合と、全対象をアノテーションした場合での学習結果、及びテスト結果を表す。 以下、それぞれのモデルを旧モデル、新モデルと表す。
2. 学習結果
旧モデルと新モデルの比較として、それぞれ同様のテストデータ(学習で使用した既知のデータ)を100枚読み込ませた結果を 以下のフォーマットで比較(新モデルに関しては同様に1000枚テストデータでテストを行って結果も追加)
・クラス正誤マトリックス
・学習時の損失の推移
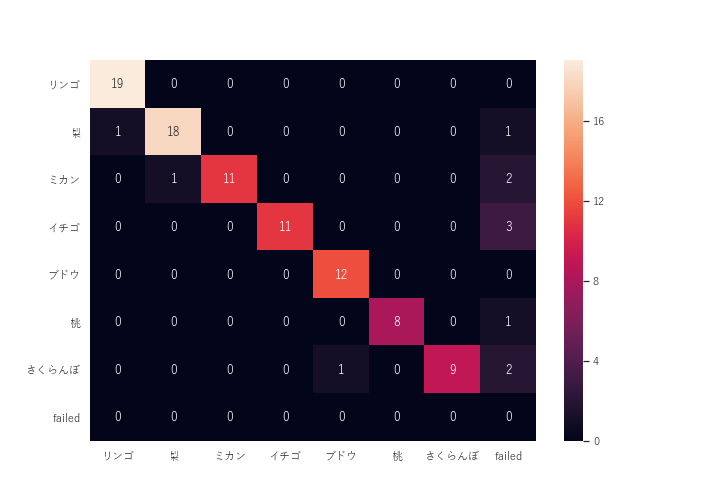
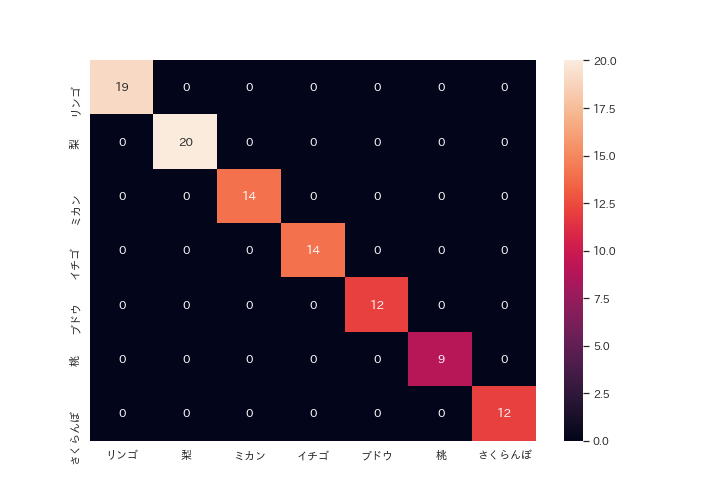
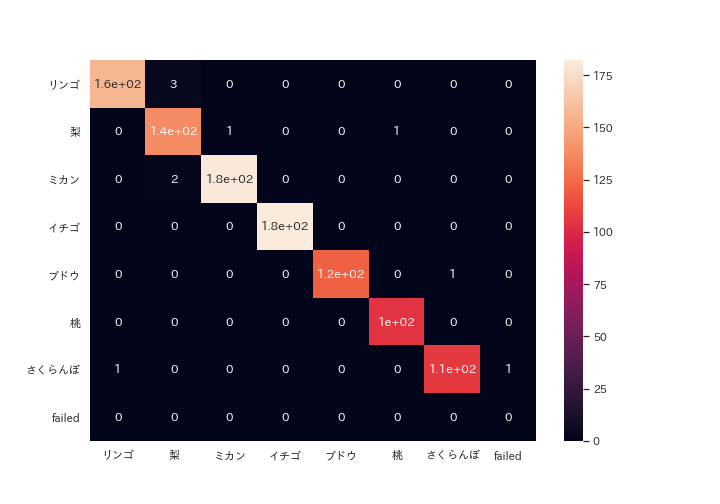
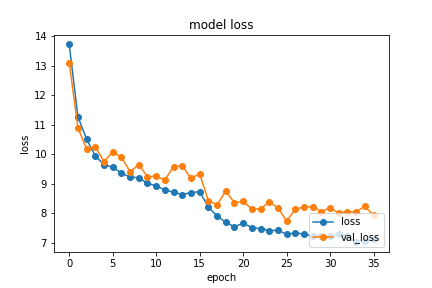
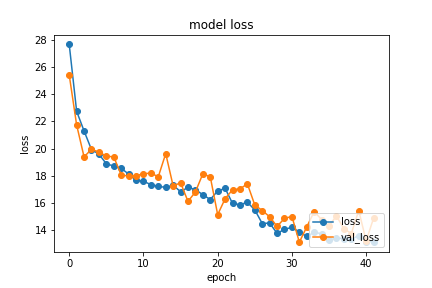
3. アノテーションを比較
旧アノテーション画像は基本的に対象を1つに絞ってアノテーションを行っている。対して、新アノテーションでは画像に写っている対象を出来る限り全てアノテーションを行っている。
例で表したように同様なアノテーションを合計1517枚行い、そのうちの9割を学習に使用し、1割を内部のテスト(今回行ったテストは別でyolo内で行っているテスト)を行った。
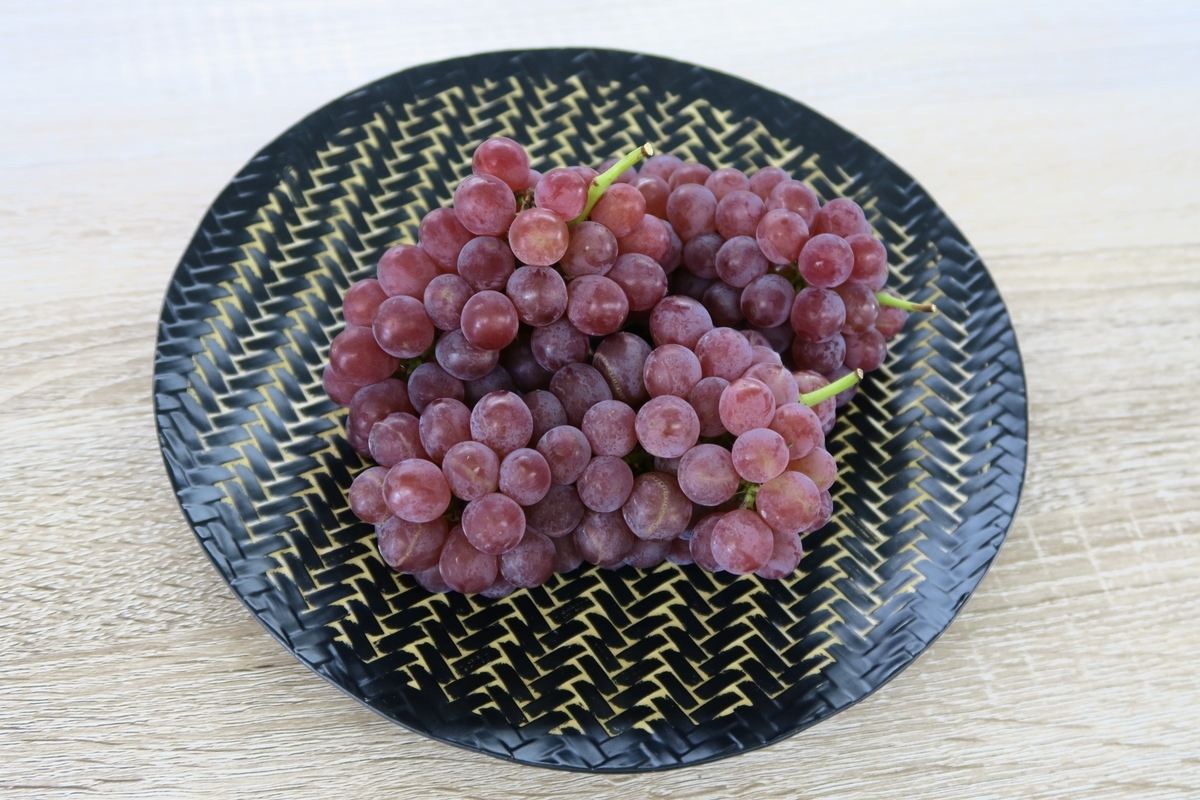
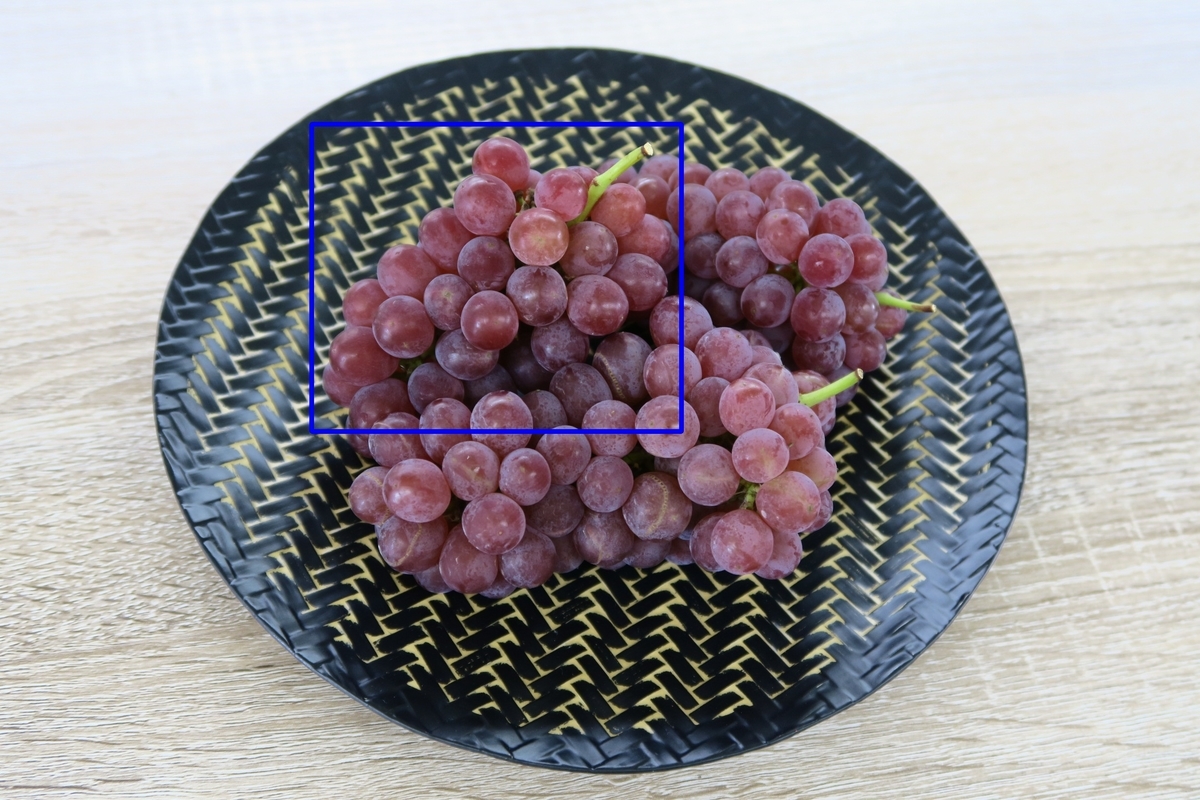
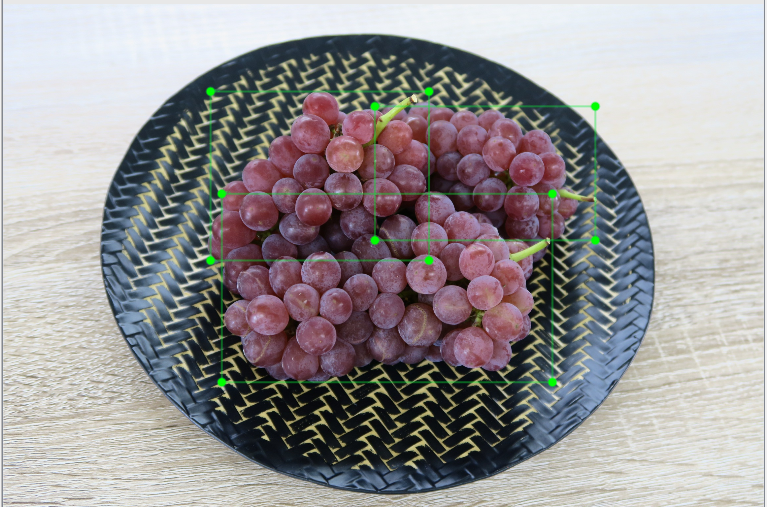
4. 認識結果
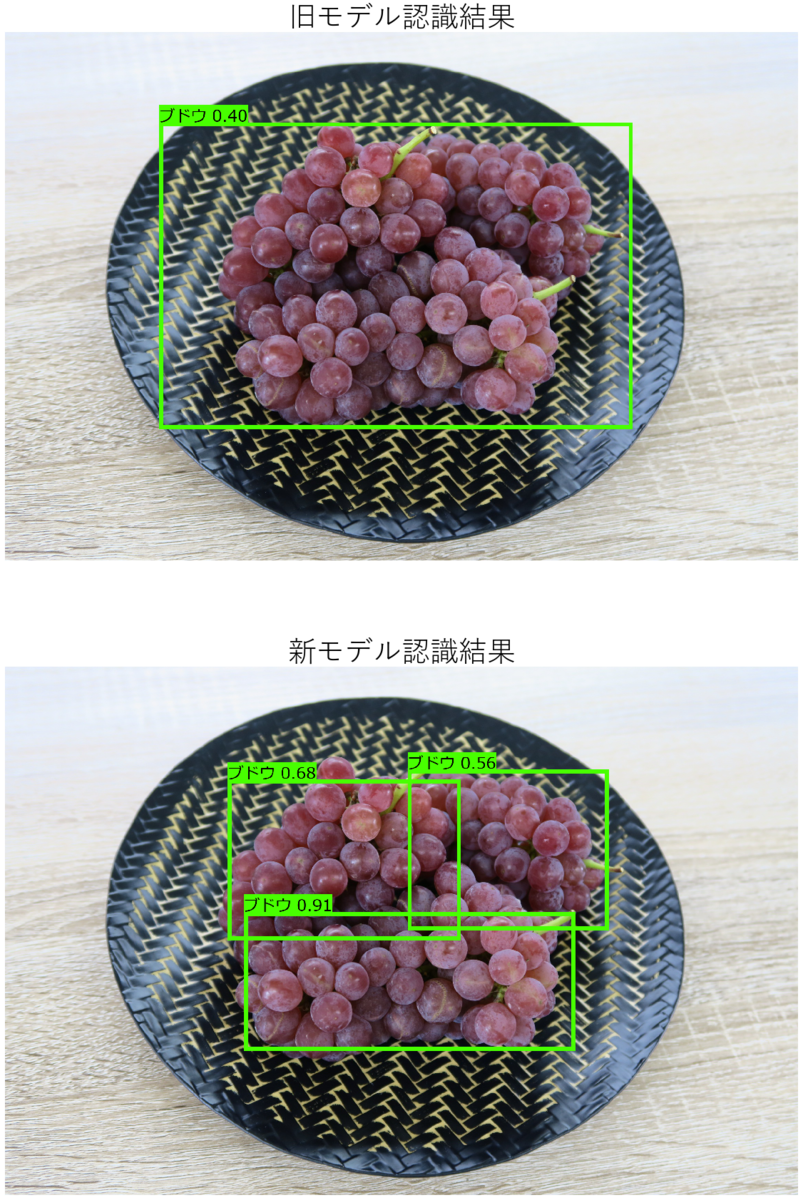
5. 考察
結果を見る限り、モデルの大幅な向上が見られたのは明白である。課題としては、未知のデータを使用したテスト結果も必要となる。(同様に100枚で実行)
また、結果から以下の2点で考えられることがある。
モデル精度と学習の損失推移の関係性
アノテーションの増減の影響
【モデル精度と学習の損失推移の関係性】
基本的に損失は低いに越したことはなく、学習の度にグラフ上で緩やかな曲線を描いて収束していくのが一般的に最適な学習を行っているといえる。その点、今回の学習は回数が一見少なく、十分に学習を行っていないように見えるが、画像認識に関しては情報量が多く、1度の学習で1000枚以上認識を行っており、他の単純な入力値の少ない学習と比べて学習回数が少ないと考える。そう考えた時に、今回の両モデルの学習は急激に損失が上がるなど過学習をしておらず、比較的最適な学習であったと言える。
そこで本題に入るが、2つの学習結果を比較した時に損失の大きさが新モデルの方が1.5倍程大きな値で収束しているのがわかる。しかし、テスト結果レポートを比較すると、新モデルの方が大幅に向上した精度が確認出来る。
故に学習時の損失が直接、モデルの精度を表すわけではないとわかる。原因として考えられるのは、1枚の画像に対するアノテーションの個数が増えたことにより、認識後の評価回数が増えたことが起因すると考えられる。
【アノテーションの増減の影響】
今回、画像の例としてブドウを認識対象として両モデルを比較したが、アノテーションの増減によって、対象の認識の仕方が変化したと考えられる。というのも、旧モデルで認識した際、ブドウ3個全体を1つのブドウと捉えて認識している。ブドウを認識すること自体は両モデル共に出来ているが、正確な認識領域の抽出に関しては、新モデルの方が見てわかるように結果が良いとわかる(ブドウの個数が合っている点)。また、領域の正確さはIOUという方式を用いて数値化することも可能であり、ある画像に対して、「何が写っている」から「どこに何が写っている」がある程度の精度で実現可能であると考えられる。
【Deep Learning】Kersa-yolo3の学習自動化
Keras-yolo3における学習リストを用いた学習の自動化を説明します。学習リストをまとめたリストを読み込ませ、1行ずつ学習を行う仕組みです。
前提として学習リストが作成済で、そのまとめリストが以下のようになります。
学習リスト/水増しデータ/調整データ/通常_上下反転_左右反転_90度回転_270度回転_上下反転左右反転/品種/6_桃/train.txt 学習リスト/水増しデータ/調整データ/通常_上下反転_左右反転_90度回転_270度回転_上下反転左右反転/品種/7_さくらんぼ/train.txt
例のパスは水増しデータ、水増しした元データ、水増し内容、種類または品種か、品種、学習リストの順になっています。
パスの構成は学習の構成に依存します。以下のスクリプトでパスを扱っている箇所を編集する必要があります。
import os, glob, shutil import numpy as np import keras.backend as K from keras.layers import Input, Lambda from keras.models import Model from keras.optimizers import Adam from keras.callbacks import TensorBoard, ModelCheckpoint, ReduceLROnPlateau, EarlyStopping from yolo3.model import preprocess_true_boxes, yolo_body, tiny_yolo_body, yolo_loss from yolo3.utils import get_random_data import datetime import matplotlib.pyplot as plt train_list_file = 'train_list.txt' train_list = [] with open(train_list_file, 'r', encoding='UTF-8') as f: line = f.readline() while line: train_list.append(line.replace("\n", "")) line = f.readline() log_path = 'logs' classes_path = 'model_data' #クラスパス anchors_path = 'model_data/yolo_anchors.txt' #これはyolo設定なのでとりあえずデフォルト first_weight = 'model_data/darknet53_weights.h5' def get_classes(classes_path): '''loads the classes''' with open(classes_path) as f: class_names = f.readlines() class_names = [c.strip() for c in class_names] return class_names def get_anchors(anchors_path): '''loads the anchors from a file''' with open(anchors_path) as f: anchors = f.readline() anchors = [float(x) for x in anchors.split(',')] return np.array(anchors).reshape(-1, 2) def create_model(input_shape, anchors, num_classes, load_pretrained=True, freeze_body=2, weights_path='model_data/yolo_weights.h5'): '''create the training model''' K.clear_session() # get a new session image_input = Input(shape=(None, None, 3)) h, w = input_shape num_anchors = len(anchors) y_true = [Input(shape=(h//{0:32, 1:16, 2:8}[l], w//{0:32, 1:16, 2:8}[l], \ num_anchors//3, num_classes+5)) for l in range(3)] model_body = yolo_body(image_input, num_anchors//3, num_classes) print('Create YOLOv3 model with {} anchors and {} classes.'.format(num_anchors, num_classes)) if load_pretrained: model_body.load_weights(weights_path, by_name=True, skip_mismatch=True) print('Load weights {}.'.format(weights_path)) if freeze_body in [1, 2]: # Freeze darknet53 body or freeze all but 3 output layers. num = (185, len(model_body.layers)-3)[freeze_body-1] for i in range(num): model_body.layers[i].trainable = False print('Freeze the first {} layers of total {} layers.'.format(num, len(model_body.layers))) model_loss = Lambda(yolo_loss, output_shape=(1,), name='yolo_loss', arguments={'anchors': anchors, 'num_classes': num_classes, 'ignore_thresh': 0.5})( [*model_body.output, *y_true]) model = Model([model_body.input, *y_true], model_loss) return model def create_tiny_model(input_shape, anchors, num_classes, load_pretrained=True, freeze_body=2, weights_path='model_data/tiny_yolo_weights.h5'): '''create the training model, for Tiny YOLOv3''' K.clear_session() # get a new session image_input = Input(shape=(None, None, 3)) h, w = input_shape num_anchors = len(anchors) y_true = [Input(shape=(h//{0:32, 1:16}[l], w//{0:32, 1:16}[l], \ num_anchors//2, num_classes+5)) for l in range(2)] model_body = tiny_yolo_body(image_input, num_anchors//2, num_classes) print('Create Tiny YOLOv3 model with {} anchors and {} classes.'.format(num_anchors, num_classes)) if load_pretrained: model_body.load_weights(weights_path, by_name=True, skip_mismatch=True) print('Load weights {}.'.format(weights_path)) if freeze_body in [1, 2]: # Freeze the darknet body or freeze all but 2 output layers. num = (20, len(model_body.layers)-2)[freeze_body-1] for i in range(num): model_body.layers[i].trainable = False print('Freeze the first {} layers of total {} layers.'.format(num, len(model_body.layers))) model_loss = Lambda(yolo_loss, output_shape=(1,), name='yolo_loss', arguments={'anchors': anchors, 'num_classes': num_classes, 'ignore_thresh': 0.7})( [*model_body.output, *y_true]) model = Model([model_body.input, *y_true], model_loss) return model def data_generator(annotation_lines, batch_size, input_shape, anchors, num_classes): '''data generator for fit_generator''' n = len(annotation_lines) i = 0 while True: image_data = [] box_data = [] for b in range(batch_size): if i==0: np.random.shuffle(annotation_lines) image, box = get_random_data(annotation_lines[i], input_shape, random=True) image_data.append(image) box_data.append(box) i = (i+1) % n image_data = np.array(image_data) box_data = np.array(box_data) y_true = preprocess_true_boxes(box_data, input_shape, anchors, num_classes) yield [image_data, *y_true], np.zeros(batch_size) def data_generator_wrapper(annotation_lines, batch_size, input_shape, anchors, num_classes): n = len(annotation_lines) if n==0 or batch_size<=0: return None return data_generator(annotation_lines, batch_size, input_shape, anchors, num_classes) def save_history(history, path): plt.plot(history.history['loss'],"o-",label="loss") plt.plot(history.history['val_loss'],"o-",label="val_loss") plt.title('model loss') plt.xlabel('epoch') plt.ylabel('loss') plt.legend(loc='lower right') #plt.tight_layout() plt.savefig(os.path.join(path, 'history.png')) plt.show() for train in train_list: """ make log folder """ if not os.path.isfile(train): print("train file does not exist:", train) exit(1) target_type = train.split("/")[-2] if target_type == "種類": classes_file = os.path.join(classes_path, target_type, "class.txt") train_type = train.split("/")[-3] train_source = train.split("/")[-4] log_dir = os.path.join(log_path, train_source, train_type, target_type) else: veriety = train.split("/")[-2] target_type = train.split("/")[-3] train_type = train.split("/")[-4] train_source = train.split("/")[-5] addition = train.split("/")[-6] classes_file = os.path.join(classes_path, target_type, veriety, "class.txt") log_dir = os.path.join(log_path, addition, train_source, train_type, target_type, veriety) if not os.path.isdir(log_dir): os.makedirs(log_dir) print("log folder made:", log_dir) shutil.copyfile(train, os.path.join(log_dir, "train.txt")) print("copy", train, "to", os.path.join(log_dir, "train.txt")) shutil.copyfile(classes_file, os.path.join(log_dir, "class.txt")) print("copy", classes_file, "to", os.path.join(log_dir, "class.txt")) """ training preparetion """ class_names = get_classes(classes_file) num_classes = len(class_names) anchors = get_anchors(anchors_path) # 画像のサイズを416x416とする input_shape = (416,416) # multiple of 32, hw # モデルのインスタンス作成 model = create_model(input_shape, anchors, num_classes, freeze_body=2, weights_path=first_weight) # make sure you know what you freeze logging = TensorBoard(log_dir=log_dir) checkpoint = ModelCheckpoint(os.path.join(log_dir, 'ep{epoch:03d}-loss{loss:.3f}-val_loss{val_loss:.3f}.h5'), monitor='val_loss', save_weights_only=True, save_best_only=True, period=3) reduce_lr = ReduceLROnPlateau(monitor='val_loss', factor=0.1, patience=3, verbose=1) # ある条件で学習をストップさせる設定 early_stopping = EarlyStopping(monitor='val_loss', min_delta=0, patience=10, verbose=1) # 訓練データと検証データに分けるとこ(とりあえずランダムで9:1) val_split = 0.1 with open(train) as f: lines = f.readlines() np.random.seed(10101) np.random.shuffle(lines) np.random.seed(None) num_val = int(len(lines)*val_split) num_train = len(lines) - num_val """ training """ # Train with frozen layers first, to get a stable loss. # Adjust num epochs to your dataset. This step is enough to obtain a not bad model. if True: model.compile(optimizer=Adam(lr=1e-3), loss={ # use custom yolo_loss Lambda layer. 'yolo_loss': lambda y_true, y_pred: y_pred}) batch_size = 8 print('Train on {} samples, val on {} samples, with batch size {}.'.format(num_train, num_val, batch_size)) model.fit_generator(data_generator_wrapper(lines[:num_train], batch_size, input_shape, anchors, num_classes), steps_per_epoch=max(1, num_train//batch_size), validation_data=data_generator_wrapper(lines[num_train:], batch_size, input_shape, anchors, num_classes), validation_steps=max(1, num_val//batch_size), epochs=10, initial_epoch=0, callbacks=[logging, checkpoint]) model.save_weights(os.path.join(log_dir, 'trained_weights_stage_1.h5')) # Unfreeze and continue training, to fine-tune. # Train longer if the result is not good. if True: for i in range(len(model.layers)): model.layers[i].trainable = True model.compile(optimizer=Adam(lr=1e-4), loss={'yolo_loss': lambda y_true, y_pred: y_pred}) # recompile to apply the change print('Unfreeze all of the layers.') batch_size = 8 # note that more GPU memory is required after unfreezing the body print('Train on {} samples, val on {} samples, with batch size {}.'.format(num_train, num_val, batch_size)) history = model.fit_generator(data_generator_wrapper(lines[:num_train], batch_size, input_shape, anchors, num_classes), steps_per_epoch=max(1, num_train//batch_size), validation_data=data_generator_wrapper(lines[num_train:], batch_size, input_shape, anchors, num_classes), validation_steps=max(1, num_val//batch_size), epochs=100, initial_epoch=10, callbacks=[logging, checkpoint, reduce_lr, early_stopping]) model.save_weights(os.path.join(log_dir, 'trained_weights_final.h5')) save_history(history, log_dir) # Further training if needed. del model K.clear_session()
最近ネタ切れです。今年はAI学習とAWSを突き進むか悩み物です。資格取得もあり、時間が許すなら他の分野にも手を出したいです。